작년 몇 달 동안, 일리노이 대학교의 물리학 자, Urbana-Champaign의 한 쌍의 물리학자인 Nigel Goldenfeld와 Sergei Maslov는 주정부의 Covid-19 Pandemic 응답에서 유명인이 없었습니다. 즉, 모든 것이 잘못 될 때까지
.그들의 전형적인 연구 분야에는 역학 또는 공중 보건이 아닌 응축 물질 물리학, 바이러스 진화 및 인구 역학을위한 모델 구축이 포함됩니다. 그러나 다양한 분야의 많은 과학자들과 마찬가지로, 그들은 미국의 반응이 실제 국가 방향이나 확립 된 테스트 프로토콜이 없을 때“데크에 대한 모든 핸드”활동의 회오리 바람이었을 때 3 월에 Covid-19 모델링 노력에 합류했습니다. 이 나라의 지도자들로부터 지역 공무원에 이르기까지 모든 사람들은 분화하는 유행성 저지방에 대처하는 데 도움이 될 수있는 모델을 요구하고있었습니다. 그들이 얼마나 나쁜지, 얼마나 빨리, 그리고 재난에서 벗어나기 위해해야 할 일을 정확하게 말할 수있는 무언가를 바라고 있습니다.
.2020 년 초에 Goldenfeld와 Maslov는 Covid-19 모델링 작업에 대한 긍정적 인 언론 보도로 샤워를했습니다. 그들의 모델은 대학이 봄에 캠퍼스를 신속하게 폐쇄하고 온라인 전용 교육으로 전환하도록 촉구하는 데 도움이되었습니다. 그 후 얼마 지나지 않아 그들의 결과는 그들의 결과를 일리노이 주지사 사무실에보고하기 위해 채용 된 여러 모델링 그룹 중 하나였습니다.
따라서 Goldenfeld, Maslov와 나머지 연구팀은 대학의 재개 과정을 안내하기위한 새로운 모델을 구축했을 때, 신뢰도가 높아졌습니다. 그들은 학생들이 분류 된 위치에서 공부, 식사, 휴식, 파티 등을 상호 작용할 수있는 다양한 방법을 설명했습니다. 그들은 캠퍼스 내 테스트 및 격리 서비스가 얼마나 잘 작동하는지 추정했습니다. 그들은 바이러스를 퍼 뜨리는 동안 학생 단체의 비율이 증상을 나타내지 않을 수있는 몇 퍼센트를 고려했습니다. 이러한 모든 요소와 그 이상에 대해, 그들은 잠재적 인 불확실성과 가상 시나리오의 광범위한 범위에 쿠션을 가졌다. 그들은 에어로졸 스프레드의 물리학을 대표함으로써 대부분의 학교 재개구 모델이 가지고 있지 않은 추가 세부 층을 내장했습니다. 교실에서 마스크를 통해 대화 할 때 또는 혼잡 한 바에서 음악을 마시고 소리를지를 때 학생이 방출 할 수있는 바이러스 입자 수는 몇 개입니다.
.모델의 지침에 따라 일리노이 대학교는 계획을 수립했습니다. 그것은 일주일에 두 번 코로나 바이러스에 대한 모든 학생들을 테스트하고, 마스크를 사용해야하며, 효과적인 연락처 트레이싱 시스템 및 노출 통지 전화 앱을 포함한 다른 물류 고려 사항 및 컨트롤을 구현할 것입니다. 수학은 이러한 정책 조합이 바이러스의 지수 확산을 터치하지 않고 직접 지시를 재개 할 수있을 것으로 제안했다.
.그러나 가을 학기에 불과 1 주일 9 월 3 일, 대학은 황량한 현실에 직면했습니다. 약 800 명의 학생들이 코로나 바이러스에 대해 긍정적 인 테스트를 받았습니다. 관리자는 비 필수 활동의 즉각적인 캠퍼스 전체 정지를 발행해야했습니다.
무엇이 잘못 되었습니까? 과학자들은 오류의 여지가 너무 많아서 학생들이 어떻게 행동 할 수 있는지에 대한 많은 우발 사태를 포함 시켰습니다. Goldenfeld는“우리가 기대하지 않은 것은 법을 어기는 것이라는 것이 었습니다. 이것은 중요한 것으로 판명되었습니다. Covid-19가 확산되는 방법을 감안할 때, 소수의 학생들만이 규칙에 반대하더라도 감염률이 폭발 할 수 있습니다.
비평가들은 Goldenfeld와 Maslov를 빠르게 공격하여 오만 혐의로 비난하고 자신의 차선에 머무르지 못한다고 비난했습니다.“우리의 방어에 갇힌 전문가들이 인정받는 사람들이 우리의 방어에 갇혀있을 때에도 이길 수 없었습니다.”라고 Maslov는 말했습니다.
한편, 일리노이 대학교는 독특하지 않았습니다. 전국의 많은 대학들은 자신의 모델이 말한 것과 실제로 일어난 일 사이의 비슷한 차이로 인정해야했습니다.
이러한 사건은 가혹한 현실을 강조했습니다. 역학적 모델만큼 엄청나게 유용하고 중요하기 때문에, 그들은 사용하는 데이터와 그들이 구축 된 가정에 민감한 불완전한 도구입니다. 그리고 그 민감성 때문에, 그들의 의도 된 목표와 사용은 종종 오해를받습니다.
이러한 모델을 개발하는 연구원들은 틀림없이 어려운 지형을 탐색해야하며, 자주없는 곳에 답을 제공해야합니다. 그들은 완전히 이해되는 바이러스의 생물학뿐만 아니라 인간 행동에 대해서는 더 미끄럽고 애매할 수있는 가정을 만들어야합니다. 그리고 그들은 정확도를 희생하지 않고 며칠 만에 워프 속도로 모든 것을 며칠 동안 분석해야합니다. 그동안 그들은 오랜 시간, 잠들지 않는 밤과 과학자, 의사 소통 자, 옹호자 및 고문으로서의 개인적인 피해를 입고 있습니다. (평범한 책임은 말할 것도없이 위기에 의해 많은 것을 확대했습니다.이 과학자들과의 전화에서 유아가 울고, 개가 짖거나, 배경에서 다른 활동에 대한 화려 함을들을 가능성이 높습니다.)
.볼더의 콜로라도 대학교 생물 학자 인 다니엘 라 레메 모어 (Daniel Larremore)는“지금은 정말 피곤하다”고 말했다. “최고의 솔루션은 없으며 사람들은 병에 걸릴 것입니다. 그리고 동료 중 한 명이 정말로 아프거나 누군가가 죽을 때 기분이 어떤지 궁금합니다.” 그는 같은 입장에있는 그와 같은 위치에있는 다른 사람들이 트위터에서 그 피로에 고백 한 후에, 누군가가 국가 안보와 방어 분야에서 일하는 사람들에게는 새로운 지분이 없다는 것을 반박했지만, 그것은 잔인한 선택에 직면하고 그들 사이에서 가장 편협한 사람들을 찾아야하는 데 도움이되지 않는다는 것을 기억합니다.
."당신은 당신이 손에 들고있는 것과 함께 최선을 다하지만 모르겠습니다."라고 그는 말했습니다. “매일 오는 숫자를보고 궁금해… 우리가 무엇을 놓치고 있는지.”
Covid-19의 글로벌 보건 위기에서 역학 모델링은 과학자, 의사 결정자 및 대중 모두에게 전례없는 역할을 해왔습니다. 불과 1 년 전,“재생 번호”및“연속 간격”과 같은 용어는 평범한 사람에게는 일반적인 문구였습니다. 과학계에서도 감염성 질병 모델링은“예쁜 틈새 연구 분야”라고 Northeastern University의 네트워크 과학자이자 역학 학자 인 Samuel Scarpino는 말했다.
지난 한 해 동안 모델은 코로나 바이러스의 내부 작업과 그것이 어떻게 퍼지는 지에 대한 창을 열었습니다. 그들은 무수한 환경에서 주어진 순간에서 질병의 영향을 보여주는 잘 초점을 맞춘 스냅 샷을 제공했으며, 그러한 상황이 어떻게 변할 수 있는지 제안했습니다. 그들은 사회의 일부를 폐쇄하고 백업하기위한 결정과 정책을 안내했습니다.
동시에, 과학자들은 모델의 한계를 도구로 인정해야했으며, 전염병이 모델의 유용성을 브레이킹 포인트로 밀어 넣을 수 있다는 실현으로. 사회에서의 질병의 황폐화는 편견이없고 일관된 환자 데이터를 얻는 데 관련된 두통을 강화시키고 모델이 반영 해야하는 인간 행동의 풍부함과 불합리성을 증폭시킵니다. 아마도 모든 사람의 가장 큰 도전은 의사 결정자들이 모델이 무엇인지, 말하지 않은지, 그리고 그들의 대답이 얼마나 불확실한지를 완전히 이해하도록하는 것입니다.
.그러나 이러한 도전은 또한 큰 개선을 주도하고 있습니다. 역학 모델링의 세계는“많은 새로운 사고, 새로운 방법”을 보았습니다. 오스틴 텍사스 대학의 수학적 생물학자인 Lauren Ancel Meyers는 말했습니다. "지난 6 년 동안 우리가 지난 6 개월 동안 우리가 아마도 우리가 아마도 우리가 아마도 6 년 동안 진행되었을 것임을 모험 할 것입니다."
.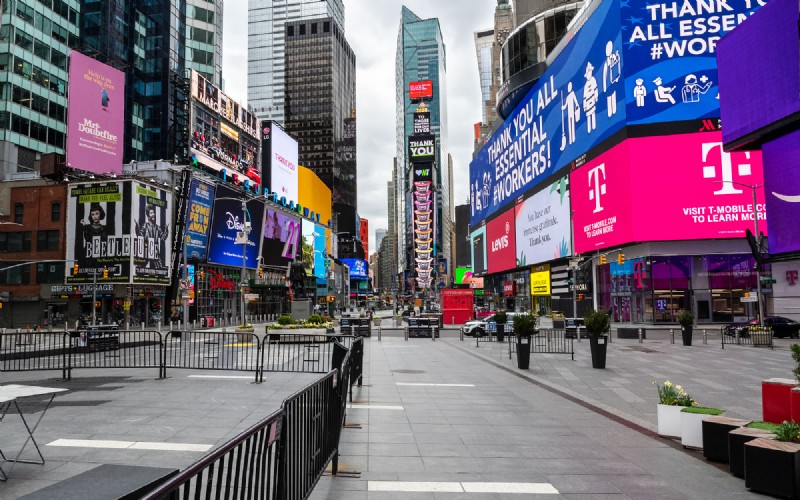
전염병 진단
전염병 전문가들이 2019 년 12 월 말과 2020 년 1 월 초에 중국에서 폐렴과 같은 질병이 자랐다는 청문을 시작했을 때, 그들은 즉시 조심하게되었습니다. 런던 위생 및 열대 의학의 역학 학자 인 아담 쿠 차르 스키 (Adam Kucharski)는“우리 분야에서”“전염병은 항상 레이더에 위협으로 레이더에있다”고 말했다. 실제로, 지난 수십 년 동안 혼자서 혼란스러워하는 4 가지 전염병 (H1N1 인플루엔자, SARS, MERS 및 에볼라)은 끊임없이 발전하고 있습니다.
그러나 중국에서 코로나 바이러스가 발생한 초기에는 많은 질병이하는 것처럼 위협에 분출 할 것인지 또는 빠르게 사라질 지 여부를 예상 할만 큼 충분히 알지 못했습니다. 바이러스가 사람에게 전염되었거나 Wuhan의 한 마켓 플레이스에서 같은 동물원에서 모든 감염이 발생 했습니까? 국경을 차단하는 데 감염이 포함되어 있거나 전 세계적으로 퍼질 수 있습니까?
사실상 데이터가없고 바이러스가 무엇을했는지 또는 어떻게 작동하는지에 대한 실제 개념이 없기 때문에 연구자들은 답변을위한 모델을 찾았습니다.
홍콩 대학교의 전염병 및 공중 보건 팀의 일원 인 수학적 질병 모델러 인 조셉 우 (Joseph Wu)는 우한 (Wuhan) 안팎에서보고 된 사례의 수를 면밀히 조사하고있었습니다. 인간과 인간 전파가 매우 가능성이 높아지기 시작했습니다. 소수의 사례는 중국의 국경을 넘어 태국, 일본에서 자랐습니다. Wu는보고 된 스프레드와 중국에서 다른 국가로의 여행량을 바탕으로 그와 그의 동료들은“Wuhan의 감염된 사례의 수는 그 당시 발표 한 것보다 훨씬 커야한다”고 말했다. 발생 크기가 훨씬 크면 사람들이 바이러스를 다른 사람들에게 전달하고 있음을 의미합니다.
Wu와 그의 팀만이 그러한 결론에 도달 한 것은 아닙니다. 2020 년 1 월 10 일, 세계 보건기구 (World Health Organization)는 유사한 분석을 수행하고 자신의 질병 모델을 모으고 같은 우려를 표명하는 전 세계 전문가 팀을위한 원격 회의를 주최했습니다.
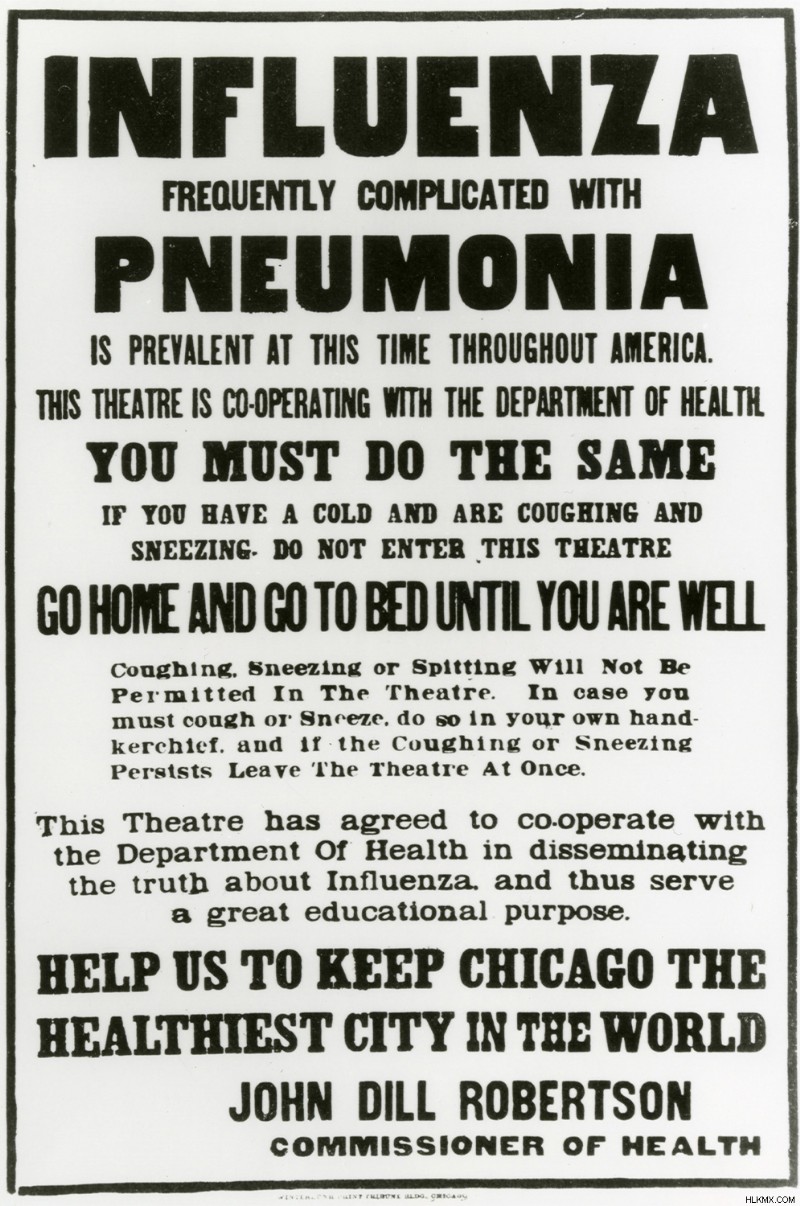
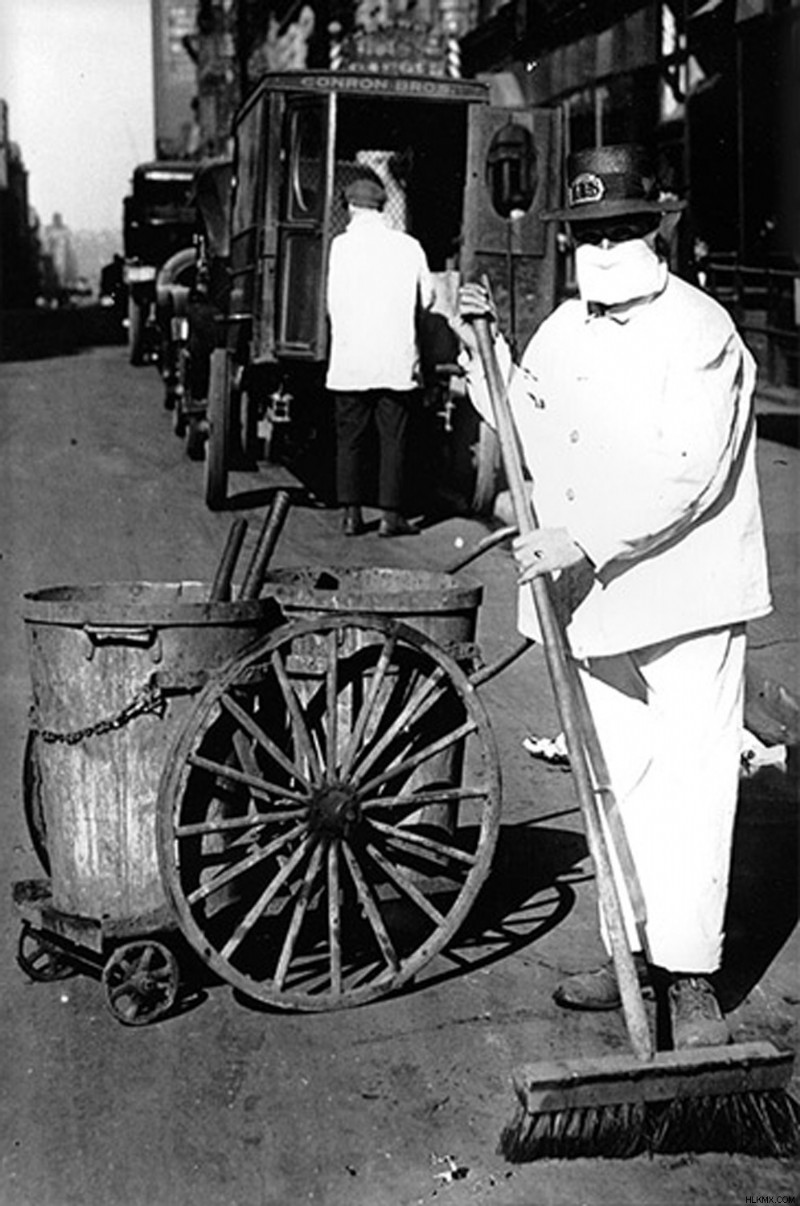
그럼에도 불구하고 홍콩 그룹은 상황을 더 잘 파악하기 위해 더 많은 데이터를 원했습니다. 2020 년 1 월 23 일, Wu와 그의 동료들은 중국 질병 통제 및 예방 센터의 회원들과 만날 계획으로 베이징으로 비행기를 탔습니다.
그들은 위기가 머리에 닿는 것처럼 그것을 만들었습니다. 그들이 공중에있는 동안 Wuhan은 공식적인 폐쇄에 들어갔다. (그들은 비행기가 착륙 한 후에 만 뉴스를 배웠다.) 지난 3 주 동안 데이터를 수집 한 중국 CDC의 연구원들과 협력 한 몇 일이 지나면 홍콩 대표단은 우한의 발병이 공식적으로보고 된 몇 천 명이 아니라 수천 건의 사례가 아니라고 결론 지었다. Wu는“우리가 데이터를보기 전에도 도시를 폐쇄하고 있다는 점을 감안할 때 우리는 이미 알고 있었다”며“우리는 이미 알고 있었다”고 말했다.
훨씬 더 관련하여, 이러한 숫자와 후속 분석은 바이러스가 빠르게 퍼질 수있을뿐만 아니라 많은 비율의 사례가 균열을 거치고 다른 곳에서 전염병을 뿌린다는 것을 암시했다. 사람들이 증상을 보이기 전에 전염의 대부분이 발생했습니다. 이것은 신속 할뿐만 아니라 적어도 부분적으로 침묵하는 유행성이라는 부름을 할 때였습니다.
Wu는“1 월 말로 다시 Wuhan이었던 1 월 말로 되감기를 상상해보십시오. "우리가 그 진술을 할 때, 약간의 압력이 있습니다. 우리가 틀렸다면 어떨까요?" 그들은 불필요하게 글로벌 공황을 일으킬 수 있습니다. 그리고 아마도 그들은 앞으로 역학적 모델이 어떻게받을 수 있는지 손상시킬 수 있으며, 앞으로 몇 년 안에 더 많은 공중 보건 위기를 조성 할 수 있습니다. 결국, 그들은 위험이“우리가 예측에 약간의 불확실성이 있다는 것을 알고 있지만 세상에 경고해야 할 충분한 위험이 있다고 결정했습니다.”라고 그는 말했다.
"연구원으로서 물론 나는 기여하기를 희망한다"고 덧붙였다. "하지만 동시에 나는 그런 책임을지는 데 익숙하지 않습니다."
unbaintable 숫자
전 세계적으로, 다른 역학 모델러들은 이러한 새로운 책임으로 어려움을 겪고있었습니다. 무증상 스프레드의 수준은 특히 받아들이고 모델링하기가 어려웠습니다. 일반적으로 호흡기 감염은 재채기와 기침, 즉 감염 증상을 통해 전달됩니다. SARS-COV-2는 더욱 비밀리에 여행했습니다. 버클리 캘리포니아 대학교의 생물 역사 학자 인 니콜라스 보석 (Nicholas Jewell)은“실제로 무증상 전염이 높은 다른 사례를 찾기 위해 어려움을 겪고 있습니다.
처음에는 Covid-19를 설명하기위한 매개 변수에 대한 지식이 거의 없기 때문에 연구자들은 코로나 바이러스로 인한 또 다른 전염병 질환 인 SARS :SARS의 숫자로 모델을 채웠습니다. 그러나 SARS의 특성을 사용하여 소설 코로나 바이러스의 전송 역학을 나타내는 것은 두 사람이 매우, 매우 다르다는 것을 보여주었습니다.
Meyers는 이것을 시도한 과학자 중 하나입니다. 그녀의 팀의 모델에서, 중국에서 나오는 초기 숫자조차도“당시 턱이 떨어지고있는 세 가지 중요한 것들을 지적했다”고 그녀는 말했다. 하나는 Wu의 팀이 보았 듯이 질병이 SARS보다 약 2 배 빠르게 퍼져 나갔다는 것입니다. 사람들이 증상을 개발하기 전에 그 확산의 상당 부분이 분명히 일어나고있었습니다. 그리고 어떤 사람들은 처음으로 증상을 느낀 후 2 주 동안 전염성을 유지할 수있는 것으로 보입니다.
메이어스가 2 월 에이 발견에 대해 미국 질병 통제 예방 센터와 다른 사람들에게 이메일을 보냈을 때, whaaat 과 같았습니다. 그녀는 회상했다. “모두는 기본적으로‘아, 당신은 틀렸어 야합니다.’라고 말했습니다. 그러나 며칠 안에 말하고 싶습니다. 갑자기 갑자기이 모든 데이터가 중국에서 추락 해 왔습니다.‘오 세상에, 이것은 정말 나쁘다. 이것은 우리가 통제 할 수없는 전염병을위한 제작물이 있습니다.””
한편, 다른 전문가들은 더 많은 도전에 대한 포즈를보고있었습니다.
런던 위생 및 열대 의학의 전염병 모델러 인 샘 애보트 (Sam Abbott)는 2019 년 가을에 박사 학위를 취득했으며 2020 년 1 월 6 일에 새로운 연구 작업을 시작하여 실시간 발생 분석을위한 통계적 방법을 개발했습니다. 그의 첫 번째 프로젝트는 예멘에서 콜레라의 발발에 중점을 두어야했지만 6 일 후에 그는 Covid-19에서 일하는 것을 발견했습니다.
.애보트는 특정 통계 기술을 사용하여 질병의 전염에 관한 요인을 추정하는 데 익숙했습니다. 그러나 그와 그의 동료들은 점차적으로 문제를 일으켰다. Covid-19의 무증상 및 상징적 전파에 대한 축적 증거는 그러한 기술이 항상 작동하지 않는다는 것을 의미했다. 예를 들어, 질병의 효과적인 생식 수는 발생하는 동안 주어진 시간에 단일 사례에 감염된 개인의 평균 수입니다 (시간이 지남에 따라이 수는 변경 될 수 있음). 그러나 많은 감염 사례가 사실상 눈에 보이지 않는다면, 연구원들은 재생산 수를 추론하는 방법이 신뢰할 수 없게되었다.
애보트는“매우 미묘한 문제이기 때문에이 문제는 즉시 과학자들에게 넘어지지 않았다”고 말했다. "단지 그것에 대해 생각하고 사람들이 서로 그래프를 보내는 데 시간이 걸렸습니다." 그러나 발견의 의미는 적어도 일부 상황에서는 연구원들이 필요한 변수를 해결하고 유용한 모델을 구축하기 위해 완전히 새로운 접근법이 필요하다는 것입니다.
.그럼에도 불구하고, 불완전한 모델과 불완전한 데이터에도 불구하고 SARS-Cov-2가 국경을 빠르게 교차하고 스프레드가 통제 불능 상태라는 것이 분명했습니다. 이 질병이 2 월 워싱턴 주 요양원을 통해 미국의 위기의 시작을 시작했을 때,“우리가 우리가하고있는 모든 일을 버려야한다는 것을 알고 있었던 순간이었다”고 전염병 문제를 연구하는 시카고 대학의 생태학 후 연구원 인 Katelyn GoStic은 말했다. 선별 여행자들은이 바이러스에 대해 완전히 비효율적 인 것으로 판명되었는데,이 바이러스는 갑자기 어디에나있는 것처럼 보였고, 과학자들은“우리 앞에 불을 내놓으려고 노력했다”고 Larremore는 말했다.
2020 년 3 월에 공식적으로 Covid-19를 발음하는 사람에게 떨어졌다. 모델링 작업은 첫 번째 중요한 역할 중 하나 인 다음과 같이 위협이 있었음을 확립하고 그 위협의 본질에 대한 세부 사항을 채우고있다.
.그리고 그 일이 일어 났을 때, 잠재적으로 수백만의 균형을 잡으면 서 정책 입안자들과 대중은 그 모델들로부터 더 많은 답변을 요구하기 시작했습니다. 다음에 일어날 일, 그리고 사회가 어떻게 반응 해야하는지에 대한 답변 - 모델이 종종 사람들이 절망적으로 원했던 형태로 제공 할 수있는 위치에 있지 않다는 답변.
.크리스탈 볼이 아님
역학적 모델은 미래에 대한 진술을하기 때문에 일기 예보에 비유하고 싶은 유혹이 있지만 심도 잘못입니다. 모델과 함께 일하는 과학자들이 신속하게 강조하기 때문에 두 사람은 결코 비교할 수 없습니다. 그러나 비슷한 종류의 예측을 할 수 있다는 잘못된 믿음은 종종 모델링의“실패”에 대한 대중의 긴장의 핵심입니다.
.일기 예보의 품질은 수천의 기상 불확실성에도 불구하고 내일 폭풍이 있을지 (그리고 얼마나 오래 지속될 것인지, 얼마나 많은 비가 올 수 있는지)를 정확하고 안정적으로 예측합니다. 그러나 기상 학자들이 허리케인의 길을 예측할 때,이 지역의 사람들이 대피하거나 머무르는 결정은 허리케인이 어디로 가는지 또는 얼마나 강한 지에 영향을 미치지 않습니다.
대조적으로, 사람들의 행동은 질병 전염에 직접적인 영향을 미칩니다. 사람들이 위협에 대응하는 방법에 대한 추가 불확실성 수준은 인간 행동, 모델링 결과 및 발발의 역학 사이의 피드백 루프를 복잡하게 만듭니다.
그렇기 때문에 역학뿐만 아니라 물리학, 생태학, 기후학, 경제 및 기타 모든 분야에서 과학자들은 미래의 오라클로 모델을 구축하지 않습니다. Kucharski는“모델은“특정 프로세스 나 우리가 관심있는 특정 과정을 이해하는 방법 일뿐입니다. 많은 역학 연구자들은 질병에 대한 유용한 통찰력을 얻는 것을 고려하고 모델링의 가장 중요한 목적이라고 생각합니다.
골든 펠트 (Goldenfeld)는 일리노이 대학교 (University of Illinois)에 대한 그의 그룹의 재개 작업에서 쫓겨 난 일부 경멸에 대한 대중의 오해를 비난합니다. “우리 모델의 목적은 [주어진 개입]이 효과가 있는지 확인하는 것이 었습니다. 2020 년 11 월 17 일에 예측되지 않았으며 234 건의 사례가있을 것입니다.”라고 그는 말했습니다. “요점은 트렌드가 무엇인지 이해하는 것입니다. 그것이 합리적으로 기대할 수있는 유일한 것입니다.”
그러나 그 차이는 특히 모델의 출력이 기만적으로 정확하게 들릴 수있는 숫자 일 때 쉽게 손실 될 수 있습니다.
그들의 핵심에서, 모든 모델은 시스템의 단순화 (반드시 간단하지는 않지만) 표현 (이 경우 인구를 통한 바이러스의 확산입니다. 바이러스의 인큐베이션 기간의 길이, 감염성을 유지하는 기간, 모든 경우에 발생하는 사망자 수 등. 모델의 알고리즘은 이러한 입력을 서로와 다른 요인과 관련하여 적절하게 조작하며 시스템의 일부 행동을 나타내는 출력을 제공합니다. 바이러스는 사례 수, 입원, 사망 또는 기타 지표에 반영된 바이러스의 확산입니다.
.전염병 및 기타 질병 발생을 이해하기 위해 과학자들은 정량적 역학적 모델링에 대한 두 가지 잘 확립 된 접근법으로 전환합니다. 각 접근 방식에는 사용 및 제한 사항이 있으며 각각은 다른 종류의 데이터로 다른 척도에서 더 잘 작동합니다. 오늘날 역학자들은 보통 두 가지를 다양한 정도로 사용하므로 모델은 둘 사이의 스펙트럼에 속합니다.
스펙트럼의 한쪽 끝에는 인구를 감수성, 감염 또는 회수 여부 (S, I 또는 R)에 따라 인구를 "구획"으로 나누고 미분 방정식 시스템을 사용하여 사람들이 한 구획에서 다른 구획으로 이동할 수있는 방법을 설명하는 질병 모델이 있습니다. COVID-19는 인큐베이션 기간이 길기 때문에-누군가가 며칠 동안 바이러스에 감염되어 다른 사람에게 전송할 수있게 될 수 있습니다. 모델은 "노출 된"구획 (E)도 포함해야합니다. 이 SEIR 모델은 일반적으로 인구가 상당히 균질하며 사람들이 비교적 고르게 섞여 모든 사람이 바이러스에 똑같이 민감하다고 가정합니다.
그러나 물론 그것은 사실이 아닙니다. 누군가의 나이, 직업, 병력, 위치 및 기타 특성은 모두 바이러스의 영향과 전송 가능성에 영향을 줄 수 있습니다. 따라서 가장 베어 본 SEIR 모델은 제한된 동작 제품군만을 나타낼 수 있습니다. 대학 재개구 계획과 같은보다 복잡하거나 전문화 된 상황을 모델링하려면 질병 테스트 및 접촉 추적과 같은 과정을 매핑 해야하는 학생, 교직원 및 교직원 간의 상세한 상호 작용 패턴이 간단한 평균에서 벗어나야합니다.
.이를 달성하기 위해 질병이 퍼지는 방식에 더 많은 구조를 추가하여 SEIR 모델을 장식 할 수 있습니다. 예를 들어, Meyers의 그룹은 상호 연결된 SEIR 모델로 구성된 거대한 모델을 구축하여 217 개의 가장 큰 도시 각각의 수십 개의 하위 집단 내에서 바이러스 전달 역학을 나타 냈습니다. 그들은 심지어 그 도시들 사이의 사람들의 움직임을 설명했습니다.
연구자들이 이러한 세부 층을 추가함에 따라, 그들은 역학 모델링 스펙트럼의 다른 쪽 끝으로 이동합니다. 대리인 기반 모델은 사람들의 그룹에 걸쳐 평균적이지 않고 상호 작용, 일상 활동 및 바이러스 자체가 감염 될 경우 바이러스 자체가 어떻게 영향을 미칠 수 있는지를 시뮬레이션합니다. (Goldenfeld와 Maslov가 일리노이 대학을 위해 개발 한 모델은 에이전트 기반이었습니다.)이 세분화는 모델이 가장 간단한 SEIR 모델에서 추상화되는 고유 한 이질성과 무작위성을 포착 할 수있게 해주었지만 비용으로 더 많은 데이터를 수집하고, 더 많은 가정을 만들고, 모델에서 훨씬 더 높은 수준의 불확실성을 관리해야합니다. 그 부담 때문에, 연구 질문이 절대적으로 요구되지 않는 한, 그들은 일반적으로 그 수준의 세부 사항을 열망하지 않습니다.
이러한 종류의 모델은 생식 수, 인큐베이션 기간 또는 무증상 스프레드 정도와 같은 매개 변수를 추론함으로써 질병에 대해 많은 것을 드러낼 수 있습니다. 그러나 그들은 또한 훨씬 더 많은 일을 할 수 있습니다. COVID-19의 경우, 이러한 모델은 무증상이거나 경미한 증상이있는 사람들이 더 아픈 것보다 전염성이 있지만 문서화 된 감염의 약 80%를 담당한다고 제안했습니다. 마찬가지로, 그들은 어린 아이들이 Covid-19를 가진 사람에게 노출되면 성인이 감염 될 가능성이 약 절반이지만,이 감수성은 10 세 동안 어린이들에게 급격히 증가한다는 것을 보여주었습니다. 사례 목록은 계속됩니다.
.이러한 가치를 유지하면 모델은 What-IF의 포트폴리오를 프로젝트 할 수 있습니다. 도시가 매장에서 잠금 조치를 해제하지만 식당이 아닌 경우 사례 수는 어떻게 될 수 있습니까? 연락처 추적 프로그램은 학교가 다시 문을 열거나 지역 병원 시스템이 압도되지 않도록하는 데 얼마나 효과적이어야합니까? 모델은 또한보다 실용적이고 즉각적인 의사 결정 지원을 지원할 수 있습니다. 질병 전염이 특정 방식으로 보이면 병원 구매가 얼마나 많은 개인 보호 장비를 구매 해야하는지, 백신의 보급이 우선 순위를 정해야합니까?
그러나 그것들은 예측이 아니라 예측입니다. 그들은 하룻밤 사이에 바꿀 수있는 가정에 시달리고 있으며, 결과적으로 그들은 불확실성으로 가득 차 있습니다.
확실히, 이러한 예상 된 미래는 여전히 매우 유용한 통찰력으로 이어질 수 있습니다. 예를 들어, Covid-19가 확산되는 방법에 대한 모델에서 Kucharski와 그의 팀은 접촉 추적만으로는 질병을 포함하기에는 충분하지 않다는 것을 알게되었습니다. 추가 조치가이를 지원해야합니다. 다른 연구에 따르면 전염병의 범위를 측정하는 것뿐만 아니라 실제 완화 측정으로 테스트가 얼마나 중요한지를 보여주었습니다. Larremore와 다른 모델의 모델은 덜 민감하지만 더 빠른 테스트가 약간 느리지 만 더 정확한 것보다 훨씬 바람직하다는 것을 나타 냈습니다. 여전히 다른 모델링 작업은 병원에 필요한 추가 직원과 침대 수를 결정하는 데 중요한 역할을했습니다.
그럼에도 불구하고, 사람들이 답을 얻기 위해 필사적 일 때 예측과 예측의 구별이 쉽게 길을 잃을 수 있습니다. 연구자들은 거의 모든 유행병에서 발생하는 것을 보았으며,이 중 첫 번째 프로젝션 모델러부터 시작하여 일반적으로 최악의 시나리오를 게시하기로 결정합니다.
.최악의 반응
3 월 중순, Imperial College London의 연구팀이 대리인 기반 모델이 영국의 Covid-19에서 최대 50 만 명이 사망했으며 전염병 과정에서 220 만 명의 사망을 예상했을 때, 사회가 문자 그대로 응답 할 때 발생할 수있는 사망자의 추정치였습니다. 그것은 가상의, 의식적으로 비현실적인 투영으로, 일어날 수있는 일의 지형을 매핑하기 시작할 수있었습니다. 그것은 결코 예측이되어서는 안된다.
그렇다면 Imperial College가 왜 출판 했습니까? 부분적으로는 개입이 얼마나 잘 작동하는지 평가하기위한 기준을 설정하는 데 도움이 되었기 때문입니다. 그러나 전 세계에서 행동을 자극했기 때문에 국가가 잠그고 다른 과감한 조치를 고려하는 데 도움이 된 모델 중 하나였습니다. Pennsylvania State University의 정량적 역학 학자 인 Matthew Ferrari는“이것은 모델의 유효한 사용”이라고 말했습니다.“펜실베니아 주립 대학의 정량적 역학 학자 인 Matthew Ferrari는“주립 폐쇄 및 국가 마스크 명령 및 물건과 같은 경보를 높이고 행동을 시작하여 미래가 지나가는 것을 막을 수 있습니다.”
.
그러나 그 아연 도금 효과는 오해의 위험이 있습니다. 존스 홉킨스 대학교 (Johns Hopkins University)의 역학 학자 인 저스틴 라일러 (Justin Lessler)는 비유대츠와의 전염병 시나리오 모델에 대해 논의 할 때 답이 종종 규모의 순서 내에만 적합하다는 것을 강조하려고한다. "나는 항상 사람들과 매우 분명하다"고 말했다. “항상 적절한 예측으로 해석됩니다. 그러나 적어도 당신은 그것을 말합니다.”
따라서 이른 봄, 영국의 사망률 통계가 임페리얼 칼리지 모델의 수치보다 덜 어둡게 보였을 때 불일치는 감각주의와 대중의 불신으로 이어졌습니다.
호주 멜버른 대학교의 수학적 생물 학자이자 역학 학자 인 제임스 맥카 (James McCaw)는“사람들은… 모델이 잘못 되었기 때문에이 모델이 잘못되었다고 말했다. “이것은 시나리오가 우리를 무서워했기 때문에 우리는 그것을 피하기 위해 응답했습니다. 모델이 잘못되었고 우리가 응답 한 것이 아닙니다.”
이런 종류의 오해는 역학에 새로운 것이 아닙니다. 서 아프리카의 2014 년 에볼라 전염병 기간 동안 미국 CDC 모델러는 발병 규모에 대한 투영에 대한 상한을 발표했습니다. 그들은 최종 표에서 12,000 명 미만의 사람들이 사망했지만 약 백만 명이 사망 할 수 있다고 제안했다. 이러한 추정치를보고 한 그룹은 아마도 발발을 감각적으로 표현한 것에 대한 격렬한 비판을 받아야했습니다. 그러나 비판은 이것이 최악의 시나리오 일 뿐이라는 사실을 무시했다.
McCaw는 그의 팀과 동료들이 호주에서 COVID-19와 자신의 상황을 모델링하기 위해 최악의 스코 나이오 추정치를 사용했을 때“그 중에서 나온 매우 무서운 숫자 중 일부”를보기 시작 했으며이 모델은 국경을 폐쇄하기로 한 국가의 결정에 큰 역할을했습니다. 사실, 호주는 초기에 전화를 걸어 엄격한 물리적 거리 조치를 신속하게 시행했으며, 사람들은 필수 활동을 관리하는 것 외에는 집을 떠나지 않아야합니다.
뒤늦게 호주는 그 현명한 선택으로부터 큰 혜택을 얻었습니다.이 나라는 행동하는 데 시간이 오래 걸리는 다른 많은 국가에서 전개 된 것보다 훨씬 작고 통제하기 쉬운 발발을 보았습니다. 대만, 한국, 싱가포르, 뉴질랜드 및 기타 지역, 특히 SARS 및 MERS 전염병 중에 배운 교훈을 적용하여 빠르고 효과적인 반응을 보였습니다. 그러나 당시 전 세계 여러 곳에서 호주가 그 일을한다고 비난했다. 나중에야, 다른 곳에서 황폐화가 명백 해졌을 때, 일부 비판은 죽었습니다.
뉴질랜드의 건강과 학업 부문 간의 협력 노력을 지휘하는 케빈 로스 (Kevin Ross)는“우리는‘우리는 5 백만 명의 사람들이 1,000 건을 위해 5 백만 명의 사람들을 폐쇄하고 있습니다.’라고 말하면서 모델을 실제로 신뢰하지 않는 사람들로 끝납니다. "그러나 그것은 100 건의 사례가 아니라 100,000 건을 피하는 것입니다."
.그들이 믿고 싶었던 이야기
불행히도, 예측과의 투영 상충은 최악의 시나리오에 국한되지 않았으며, 오해는 여론에 국한되지 않았습니다. 다른 모델링 추정치도 잘못된 방식으로 진행되었습니다.
It perhaps didn’t help that there was an over-proliferation of models from researchers far afield of epidemiology:an explosion of preprints from physicists, economists, statisticians and others who had their own extensive experience with complex modeling and wanted to help end the pandemic. “I think everybody who had a spreadsheet and had heard the words ‘S,’ ‘I’ and ‘R’ felt they should make a model,” Lessler said. “I don’t want to say none of them did a good job, but for the most part, it’s not so much about the math and the technical stuff. It’s more about understanding where you can go wrong in the assumptions.”
Scarpino agreed. “We have somehow managed to do this for every single pandemic and outbreak that ever happens,” he said of well-intended scientists entering the epidemiological modeling arena — and while that can be helpful, it also runs the risk that they might “just reinvent broken wheels.”
One of the first models to capture the ear of the White House was a statistical model published by the University of Washington’s Institute for Health Metrics and Evaluation (IHME). The IHME’s primary expertise is in analyzing the efficacy of health systems and interventions, but the organization wasn’t particularly experienced in epidemiological forecasting or infectious disease modeling as such.
As a result, their model technically qualified as epidemiological, but it didn’t take into account the virus’s mechanism of transmission or other key characteristics of the epidemic. Instead, it fit a curve to mortality data in the U.S. based on several basic premises:that the curve would take the same general shape as it did in China and Italy, where the infection rate was already declining; that people would generally comply with government-level policies and mandates; and that social distancing and other interventions would have the same impact as in China.
A key caveat, though, was that the IHME’s model relied entirely on those assumptions. If any of those conditions changed or weren’t quite right in the first place, the model’s outputs would no longer be relevant.
And many of the model’s assumptions were already not holding. People’s behavior wasn’t coupled to the implemented policies:They got scared and started social distancing long before governors announced stay-at-home orders. But “stay at home” and “social distancing” by U.S. standards also looked nothing like what was being enforced in China. While the epidemic in China followed a simple rise-and-fall progression, the U.S. was hitting a second peak before its first ended, forming a “double S” shape. Unguided by any underlying biological mechanism, the model had no way to account for that changing dynamic. As a result, its estimates fell overwhelmingly short of reality.
“Some of the original forecasts from the IHME group were using a method that had been thoroughly debunked before I probably was even born,” Scarpino said.
That might not have mattered in the long run if the model had been used properly. Typically, epidemiologists use a statistical model like the IHME’s as part of what’s known as an ensemble forecast. (Despite its name, an ensemble forecast is really like a model built from other models, and it too offers only projections, not predictions.) The IHME model’s results would be averaged and weighed mathematically with the outputs of dozens of other epidemiological models, each with its own shortcomings, to achieve the modeling equivalent of the “wisdom of the crowd.” One model in an ensemble might be better at handling unlikely events, another might excel at representing transmission when the caseload is higher, and so on; some models might be statistical, others might be SEIR types that stratify populations in different ways, still others might be agent-based.

“Consensus is not always what you’re after. You don’t want groupthink,” McCaw said. With so much uncertainty in the science and data, “it’s good to have multiple models in different perspectives.”
Comparisons among multiple models can also substitute to a degree for vetting their quality through peer review. “You can’t do peer review fast enough to help in an epidemic,” Goldenfeld said. “So you have to do peer review not sequentially … but in parallel.”
Unfortunately, Lessler said, “it took longer than it should” to get those ensembles up and running in the U.S. The lack of a coordinated national response may have been a root cause of the delay. Scientists were left to their own devices, without the resources they needed to pivot easily from their everyday work to the around-the-clock dedication that COVID-19 ended up requiring. Often, they essentially had to volunteer their time and effort without proper funding and had to establish networks of communications and collaboration as they worked. Much of the infrastructure that could have helped — a pandemic preparedness group in the White House, a centralized top-down organizational effort to connect expert modeling teams with other researchers and officials, and of course core funding — was entirely absent.
Without the ensembles, that left the IHME model, with its single perspective and other problems, as the most appealing strategic resource available to many decision-makers.
“When COVID emerged, the IHME model seemed to come out of nowhere and really got a ton of attention,” Meyers said. “It was being cited by Trump and the White House coronavirus task force, and they had a really nice, visually intuitive webpage that attracted public attention. And so I think it was really one of the most noticed and earliest forecasting models to really put forecasting on the radar in the public imagination.”
Epidemiologists grew alarmed when, in April and May, the IHME projections were used by the White House and others to say that the U.S. had passed the peak of its outbreak, and that case numbers and deaths would continue to decline. Such claims would hold true only if the U.S. stayed under lockdown.
But “people used those models [and others] to reopen their cities and justify relaxing a lot of the stay-at-home orders,” said Ellie Graeden, a researcher at the Georgetown University Center for Global Health Science and Security and the founder of a company that specializes in translating complex analysis into decision-making. “It suggested a degree of optimism that I think assuaged concern early in the event.”
Graeden thinks this made it much harder to get the public and decision-makers to heed more realistic scenarios. “It’s not that IHME was the only model out there,” she said. “It was a model that was showing people the story that they wanted to believe.”
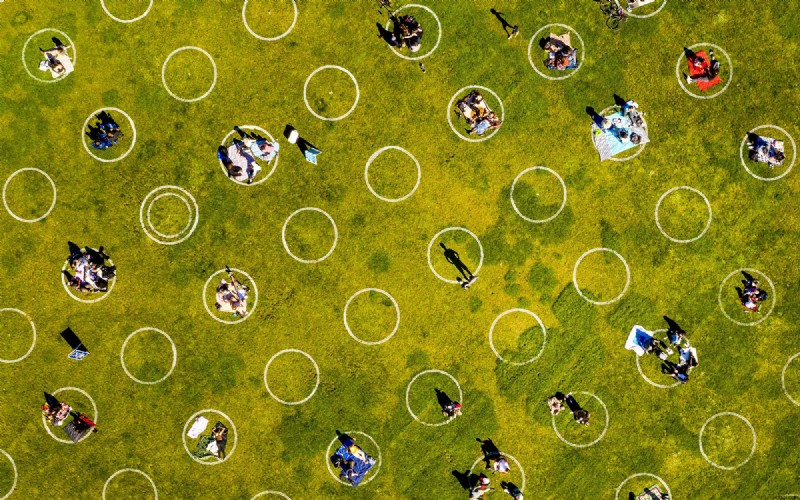
The IHME has since revised its model repeatedly, and other research teams, including Meyers’ group, have used their epidemiological experience to build on some of its core machinery and improve its projections. (Those teams have also developed their own new models from scratch.) The current version of the IHME model is one of many used in an ongoing ensemble forecasting effort run by the CDC. And the IHME has since become more transparent about its assumptions and methods — which has been crucial, given the extent to which uncertainties in those assumptions and methods can propagate through any model.
After all, even the best models are dogged by uncertainties that aren’t always easy to recognize, understand or acknowledge.
Reckoning With Uncertainty
Models that rely on fixed assumptions are not the only ones that need to be navigated with care. Even complex epidemiological models with built-in mechanisms to account for changing conditions deal in uncertainties that must be handled and communicated cautiously.
As the epidemic emerged around her in Spain, Susanna Manrubia, a systems biologist at the Spanish National Center for Biotechnology in Madrid, became increasingly concerned about how the results of various models were being publicized. “Our government was claiming, ‘We’ll be reaching the peak of the propagation by Friday,’ and then ‘No, maybe mid-next week,’” she said. “And they were all systematically wrong, as we would have expected,” because no one was paying attention to the uncertainty in the projections, which caused wild shifts with every update to the data.
“It was clear to us,” Manrubia said, “that this was not something that you could just so carelessly say.” So she set out to characterize the uncertainty rooted in the intrinsically unpredictable system that everyone was trying to model, and to determine how that uncertainty escalated throughout the modeling process.
Manrubia and her team were able to fit their models very well to past data, accurately describing the transmission dynamics of COVID-19 throughout Spain. But when they attempted to predict what would happen next, their estimates diverged considerably, sometimes in entirely contradictory ways.
Manrubia’s group was discovering a depressing reality:The peak of an epidemic could never be estimated until it happened; the same was true for the end of the epidemic. Work in other labs has similarly shown that attempting to predict plateaus in the epidemic curve over the long term is just as fruitless. One study found that researchers shouldn’t even try to estimate a peak or other landmark in a curve until the number of infections is two-thirds of the way there.
“People say, ‘I can reproduce the past; therefore, I can predict the future,’” Manrubia said. But while “these models are very illustrative of the underlying dynamics … they have no predictive power.”
The consequences of the unpredictability of those peaks have been felt. Encouraged by what seemed like downturns in the COVID-19 numbers, many regions, cities and schools reopened too early.
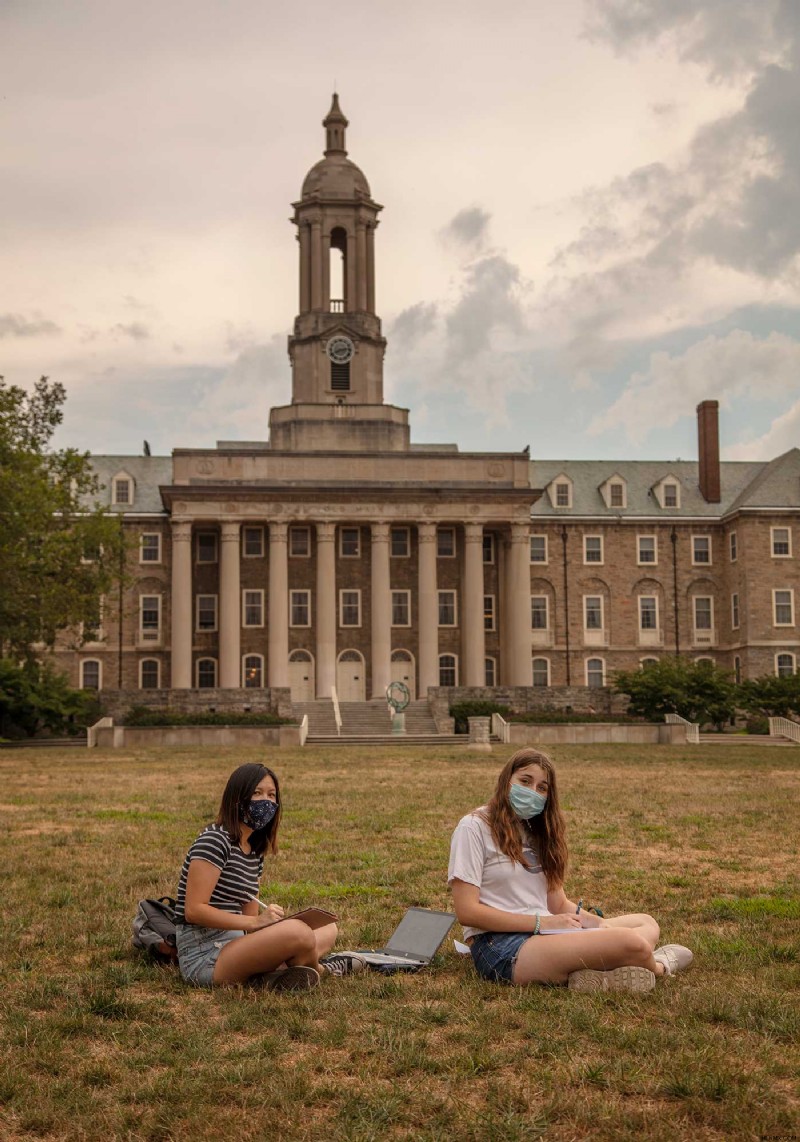
Ferrari and his colleagues at Penn State, for instance, had to confront that possibility when they started making projections in March about what August might look like, to inform their more granular planning models for bringing students back to campus. At the time, it seemed as if the first wave of infections would be past its peak and declining by the summer, so Ferrari and the rest of the modeling team assumed that their focus should be on implementing policies to head off a second wave when the students returned for the fall.
“And then the reality was, as we got closer and closer, all of a sudden we’re in June and we’re in July, and we’re all yelling, ‘Hey, the first wave’s not going to be over,’” Ferrari said. But the reopening plans were already in motion. Students were coming back to a campus where the risk might be much greater than anticipated — which left the team scrambling to find an adequate response.
Chasing the Data
An unfortunate early lesson that COVID-19 drove home to many researchers was that their modeling tools and data resources weren’t always prepared to handle a pandemic on the fly. The biggest limitations on a model’s capabilities often aren’t in its mathematical framework but in the quality of the data it uses. “The best model could not account for our lack of knowledge about epidemiology, about the biology,” Wu said. Only good data can do that.
But gathering data on a pandemic as it happens is a challenge. “It’s just an entirely different ballgame, trying to produce estimates in real time,” Gostic said, “versus doing research in what I would describe as more of a peacetime scenario.”
“It’s a war,” McCaw agreed:one waged against chaos, against inaccuracies, against inconsistencies, against getting completely and utterly overwhelmed. “It’s really hard to get the right information.”
A key number that epidemiological modelers want to know when collecting data, for instance, is the total number of infections. But that’s an unobservable quantity:Some people never visit a doctor, often because they have mild symptoms or none at all. Others want to get tested to confirm infections but can’t because of an unavailability of tests or lack of testing infrastructure. And even among those who do get tested, there are false positives and false negatives to consider. Looking at the number of reported cases is the next best option, but it’s just the tip of the iceberg.
Gathering even that data in a timely way for COVID-19 was often nearly impossible early on. “I grew up in the information age, and so I guess I naively assumed at the start of this pandemic that state departments of public health would have some sort of button, and they could just press that button and data from hospitals around the state would just automatically be routed to some database,” Gostic said. “But it turns out that that button doesn’t exist.”
Scientists who had hoped to immediately start building useful models instead spent most of February and early March just trying to gain access to data. They spent weeks cold-calling and emailing hospitals, departments of public health, other branches of government and consulting companies — anyone they could think of. Researchers had to sort through texts, faxes, case reports in foreign languages and whatever else they could get their hands on, all the while worrying about where that data was coming from and how accurate it was.
It’s been “a real disappointment and a surprise,” said John Drake, an ecologist at the University of Georgia, “that as a country or globally, we’ve done such a poor job collecting data on this epidemic. … I genuinely thought that there would be a government response that would be effective and coordinated, and we haven’t had that.”
“None of us, I think, were prepared for the inconsistent data collection,” he added.
In those early days of the epidemic, case data in the U.S. and other regions was so unreliable and unrepresentative that it often became unusable. Case counts were missing large numbers of asymptomatic and mildly symptomatic infections. Testing and reporting were so scarce and inconsistent that it distorted the numbers that researchers obtained. Pinning those numbers down in real time was further complicated by the lag between when a person got infected and when they showed up in the reported case data. Even the very definition of a “case” of COVID-19 changed over time:At first, an infection was only considered an official case if a person had traveled to Wuhan, exhibited particular symptoms, and then tested positive (the tests were different then, too). But as weeks and then months passed, the criteria kept expanding to reflect new knowledge of the disease.
For some researchers, these problems meant turning to data on hospitalizations and deaths from COVID-19. The recordkeeping for those numbers had its own shortcomings, but in some ways it was more reliable. Yet that data captured still less of the full picture of the pandemic.
“Models aren’t a substitute for data,” Kucharski said.
It wasn’t until late April or May, with the establishment of more comprehensive testing (as well as more reliable pipelines for case data), that some scientists started feeling comfortable using it. Others tried to account for the issues with case data by applying various statistical techniques to translate those numbers into something more representative of reality. As always, there was no right answer, no obvious best path.
Because of these complications, it took months to pin down good estimates for some of the key variables describing COVID-19. During that time, for example, estimates of the proportion of asymptomatic cases jumped from 20% to 50%, then back down to 30%.
Modeling groups also put out diverse estimates of the infection fatality ratio — the number of people who die for every person who gets infected, an important parameter for estimating a potential death toll. Every aspect of the calculation of that figure had huge uncertainties and variability — including that the number itself can change over time, and that it differs based on the demographics of a population.
Unfortunately, the infection fatality ratio is also a number that has been heavily politicized in a way that demonstrates “an abuse of models,” Larremore said. Factions pushing for earlier reopening, for instance, emphasized the lower estimates while disregarding the related epidemiological considerations. “People have their conclusions set a priori, and then there’s a menu of possible models, and they can look at the one that supports their conclusions.”
Part of what built researchers’ confidence in the values they were getting was the emergence of special data sets that they could use as something like an experimental control in all the chaos. For example, one of the largest known COVID-19 outbreaks in February outside of China occurred on the Diamond Princess cruise ship, docked in quarantine off the coast of Japan, where more than 700 people were infected. Over time, scientists reconstructed practically a play-by-play of who was infected when and by whom; it was as close to a case study of an outbreak as they were likely to get. The Diamond Princess event, along with similar situations where the surveillance of populations captured the spread of the disease in extraordinary detail, told researchers what they needed to know to reduce the uncertainty in their estimates of the infection fatality ratio. That in turn helped improve their models’ projections of the total number of deaths to expect.
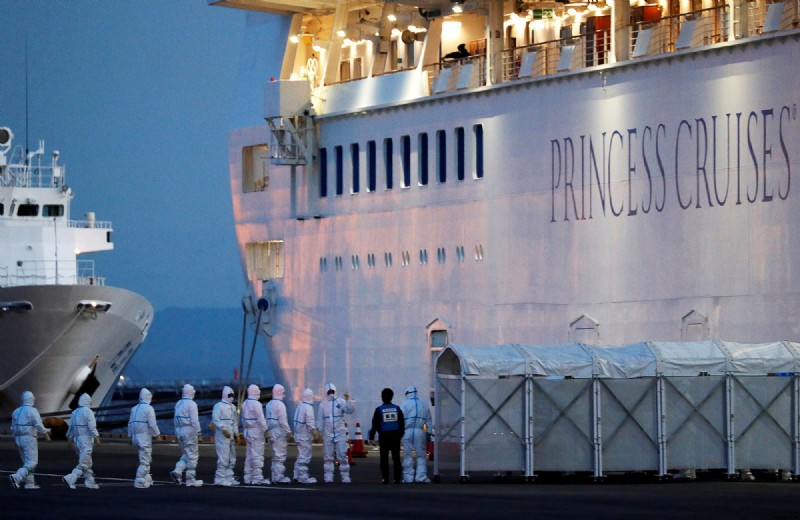
Some of the most comprehensive data came in the summer months and beyond, as testing became more prevalent, and as researchers designed serology studies to test people in a given population for antibodies. Those efforts gave a clearer snapshot of the total number of infections, and of how infection related to other factors.
But these parameters are ever-moving targets. In the U.S., for instance, researchers observed a drop in the infection fatality ratio as hospitals improved their treatments for the disease, and as changing behavior patterns in the population led to a higher proportion of infections among young people, who had a better chance of recovering. Only constant updates with high-quality data can help researchers keep up with these changes.
Obtaining good data on COVID-19 continues to be a problem, however, not just because of shortcomings in the data collection process but because of intrinsic characteristics of the virus that affect the data. Even the reproduction number has proved trickier to estimate than expected:Because COVID-19 mostly spreads through random, infrequent superspreader events, a simple average value for how quickly it’s transmitted isn’t as useful. Moreover, during past epidemics, modelers could estimate changes in the reproduction number over time from data about the onset of symptoms. But since so many COVID-19 infections occur asymptomatically or presymptomatically, symptom onset curves can be misleading. Instead, modelers need curves based on infection data — which for COVID-19 can only be inferred. This messiness makes it difficult to look back and analyze which interventions have worked best against the disease, or to draw other conclusions about apparent correlations.
The Biggest Problem Is Us
But by far, the biggest source of uncertainty in COVID-19 models is not how the virus behaves, but how people do. Getting that X-factor at least somewhat right is crucial, given just how much people’s actions drive disease transmission. But people are capricious and difficult to understand; they don’t always act rationally — and certainly not predictably.
“Modeling humans is really hard,” Graeden said. “Human behavior is idiosyncratic. It’s culture-specific,” with differences that show up not just between nations or demographics but between neighborhoods. Scarpino echoed that idea:“You walk across the street and it’s a different transmission dynamic, almost,” he said.
Ferrari and his colleagues have seen just that at Penn State. Since the fall, they’ve repeatedly conducted antibody tests on both university students and people who live and work near campus. They found that even though the outbreak infected 20%-30% of the 35,000 students, the surrounding community had very little exposure to COVID-19. Despite their proximity, the students and the townsfolk “really did experience completely different epidemic settings,” Ferrari said.
Those differences weren’t limited to behavioral or cultural practices but extended to systemic considerations, like the ability to work from home and access to resources and care. “I think most people, given the opportunity, would exhibit good individual behavior,” Ferrari said. “But many people can’t, because you first need the infrastructure to be able to do so. And I think those are the kinds of insights that we’re slowly moving towards trying to understand.”
A further complication is that past sociological studies of human behavior no longer apply:During a pandemic, people simply aren’t going to behave as they normally do.
“One of the big sort of ‘oh no’ moments,” Meyers said, “was when we realized that everything we’d been assuming about human contact patterns and human mobility patterns was basically thrown out the window when we locked down cities and sheltered in place. We didn’t have the data. We didn’t have the pre-baked models that captured this new reality that we were living in.”
Unfortunately, top-down regulations can’t be used as proxies for people’s actual behaviors. Anonymized data about people’s movements — from cell phones and GPS-based services — has shown that people mostly stopped moving around early in the pandemic, independently of whether lockdowns were in place in their region; people were scared, so they stayed at home. Meanwhile, interventions like mask mandates and bans on indoor dining were instituted but not always enforced, and people gradually moved around and interacted more as the months wore on, even as the number of deaths per day rose to unprecedented heights.
Those kinds of behaviors can significantly complicate the shapes of epidemic curves. But knowledge of those behaviors can also illuminate the deviations between what researchers observed and what they expected. For example, the modeling for the spread of COVID-19 through nursing homes and long-term care facilities didn’t initially match the observed data. Eventually, scientists realized that they also had to take into account the movement of staff members between facilities. But it took time to pinpoint that behavioral factor because such specific movements are usually abstracted away in simpler models.
“Confronting the link between behavior and transmission is difficult,” said Joshua Weitz, a biologist at the Georgia Institute of Technology, “but it has to be prioritized to improve forecasting, scenario evaluation, and ultimately to design campaigns to more effectively mitigate and control spread.”
This realization led researchers to pursue data from cell phones and other sources, to design comprehensive surveys about interactions and other activities outside and within households, and to integrate that information into epidemiological models at a massive scale. “We hadn’t actually really developed the technology to do that [before],” Meyers said, because there had been no urgent call for that data (or no access to it) before COVID-19. A new methodology was needed, along with new ways to assess the quality and representativeness of the data, which was often supplied by private companies not subject to the same scrutiny as other epidemiological data sources. “All of that we’ve developed in the last few months,” she said.
Uncertain Communications
These different types of uncertainty add up and have consequences down the line. Because small uncertainties can have exponentially bigger effects, “it’s a little bit like chaos,” Goldenfeld said. But communicating that uncertainty to decision-makers in a way that’s still useful and effective has proved a particularly difficult task.
“Decision-makers want answers,” Graeden said, but “a model cannot produce an answer. And it shouldn’t. It can get close. It can be suggestive.” To her, the pandemic has only highlighted a long-standing communications challenge. “There’s actually a culture clash here, where fundamentally the communication always will be broken, because the scientist has been trained not to give an answer, and the decision-maker’s only job is to provide one.”
Abbott recalls getting feedback from decision-makers about having too much uncertainty in some of his modeling work. “I’m not sure what the happy balance is,” he said. “You need what actually happens to be encapsulated by your estimates going forward; otherwise people lose confidence. But equally, if you’re too uncertain, people can’t make any decisions off your estimates.” When asked what he’d done since getting the feedback about reducing uncertainty, “I accidentally added more,” he said, a bit sheepishly.
One way researchers and decision-makers have tried to bridge that culture gap is to focus on qualitative narratives that emerge from all the models, rather than specific quantitative outcomes. Just knowing something as simple as whether the epidemic is growing or shrinking at a given time can be immensely helpful, even if different models spit out very different numbers to reflect it. The trend can be used to analyze which interventions have been most effective in various contexts, and to suggest which combinations of interventions might be best to try going forward.
“Trends are more robust than precise numbers, and can be used to plan responses,” Goldenfeld said. “Instead of saying, ‘Can you predict for me how many hospital beds am I going to need?’ what you can predict is, ‘What is the probability in the next, say, three weeks that the hospital capacity in this region is going to be exceeded … and by how much?’”
Relying on multiple models also allows researchers and decision-makers to address a variety of assumptions and approaches to bolster confidence in whatever conclusion they reach. Kucharski explained that if two completely different models both conclude that some level of contact tracing isn’t enough to control an outbreak, “then the difference in structure and the differences in precise estimates are less relevant than the fact that they both come to the same answer about that question.”
Even the uncertainty itself can be informative. In December, officials at Penn State, including Ferrari and his team, initially settled on reopening their campus in mid-January. But as 2020 drew to a close, they decided to push that plan back a month:Students will now be returning to campus on February 15. Because of factors such as the rise in levels of transmission in the surrounding county, there was “a real concern that we just wouldn’t be able to maintain operations at the level that was necessary to bring students back,” Ferrari said.
But the decision was also based on uncertainties in the projections of a model that Ferrari and his colleagues had been looking to for guidance — the CDC’s ensemble forecast, which aggregates the results of dozens of individual models. In December, the bounds on the uncertainties were so wide that “we didn’t really quite know what January was going to look like,” he said.
He and his team also noticed that many of the individual models in the ensemble were under-predicting the number of cases that would arise. “For the three to four weeks before that decision got made, we were seeing in reality worse outcomes than the models were projecting,” Ferrari said. “So that gave us pause and made us think that really, the coming three to four weeks are more likely to be on the pessimistic side of that confidence bound. And we just really couldn’t accept that.”
The trustworthiness of the models isn’t the only consideration in setting policy. According to McCaw, it also helps to have cultivated relationships with government officials and other policy-setters. He and members of his team have spent 15 years doing this in Australia, mostly through discussions about how best to respond to flu epidemics. “We’ve had hundreds and hundreds of meetings now,” he said. “We learn each other’s styles, we learn each other’s quirks, and we have a lot of trust.” Those long-term relationships have helped him figure out how to explain his work in a way that not only makes sense to whoever he’s interacting with, but also allows them to explain it to other policy leaders.
In line with that goal, over the past five years and more, McCaw and his colleagues have conducted workshops and programs to figure out how best to visualize and communicate their modeling. (Tables of numbers work surprisingly well. Though they “can feel a bit overwhelming and busy,” according to McCaw, “they’re a high-dimensional visualization of something, so they’re actually quite powerful.” Heat maps are less successful for anything other than geographical data. Graphs have their uses, but they need to be directed at a particular policy question.) Most importantly, the Australian team learned that “shying away from uncertainty is a disaster,” McCaw said. “So we embraced that.”
Other researchers have gone straight to social media instead, posting their preprints and models on Twitter to inform the public directly and to gain access to academics and government officials through less formal channels.
These forms of communication remain difficult and time-consuming, and many researchers have had to learn about them on the fly as the pandemic progressed. “It’s really made me wish that there were more communication lessons in STEM education,” said Kate Bubar, a graduate student in Larremore’s lab at the University of Colorado, Boulder who started pursuing her doctorate during the COVID-19 crisis.
The exchanges with policy-setters also benefit the scientists by illuminating decision-making processes, which can seem opaque when they go beyond the models’ math to consider other factors.
When case numbers at Penn State shot up after its reopening, Ferrari and others at first urged university officials to close the campus again immediately. But then, he recalls, the head of university housing told him, “Matt, do you understand what ‘shut it all down, send them all home’ means?” He pointed out that the school’s 40,000 students would not suddenly disperse; 30,000 of them would likely stay in the apartments they had rented. Moreover, the logistics of getting everyone off campus were intimidatingly hard and could even worsen the risk of spreading COVID-19.
“And I think each one of us went through cases like that,” Ferrari said, “where we got to the end of a really impassioned discussion and said, ‘OK, you know, I’m going to stand down because now that I can see the case from your perspective, I recognize all of the things that I was glossing over.’”
How Models Can Help Next
Epidemiological models will continue to be crucial in what everyone hopes will be the end stages of the pandemic, particularly as researchers look into the next big questions, such as how to prioritize the dissemination of vaccines. A common way to do so for the flu, for instance, is to target vaccines at children, which indirectly protects the rest of the population because kids are a major node in the network of flu transmission (though they do not seem to be for COVID-19).
Larremore and Bubar have been part of the ongoing effort to model what that should look like for COVID-19. Long before any vaccines were available, they considered an extensive list of uncertainties:about the disease dynamics, about whether a vaccine would block disease transmission, about how long immunity might last, and other factors. A big question was how effective a vaccine needed to be, and in what way that efficacy would manifest:If a vaccine was 50% effective, did that mean it worked perfectly but only in 5 out of 10 people, or that it cut everyone’s chances of infection in half?
They quickly found that in setting COVID-19 vaccination strategies, it’s necessary to choose between the goals of reducing deaths and reducing transmission. If they wanted to minimize mortality, direct vaccination of the elderly was the way to go, regardless of vaccine efficacy and other what-ifs. But if the goal was instead to reduce transmission (in places with large numbers of essential workers who couldn’t stay in lockdown, for instance), then adults between the ages of 20 and 50 should be vaccinated first instead:Their behaviors and interactions made them more of a hub for further spread. In that case, however, there would also be slight differences in allocation based on the efficacy of different vaccines, population demographics and contact patterns. “We got different results for Brazil, Belgium, Zimbabwe and the U.S.,” Larremore said.
Now that multiple vaccines have been approved in various countries around the world, researchers have been able to refine those models. But they’ve also had to expand on their work to account for new events that are shifting priorities again. One is the emergence of new SARS-CoV-2 mutations that have raised the transmission rate in many regions. Another is that the rollout of vaccines is happening much, much more slowly than had been anticipated.
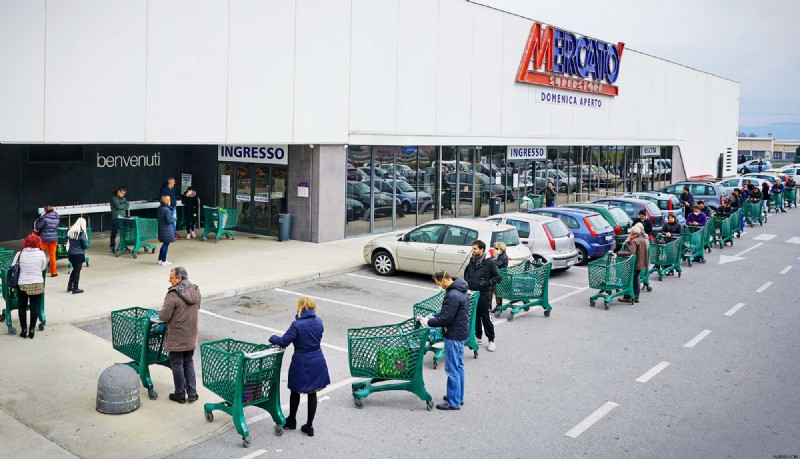
Still, to Larremore, the basic calculus stays the same. “It’s a race,” he said. “It’s racing against the virus itself that’s hopping from person to person.” And so, “as you roll the vaccine out more and more slowly, it’s going to change what strategy you would use.”
In countries where rollout has been very slow compared to viral transmission, the models show that taking the direct approach and reducing mortality — by first vaccinating older people and others who are at higher risk — is the best way to move forward. But “if you’re South Korea, Taiwan, New Zealand” or somewhere else where transmission is under better control, Larremore said, “you have a totally different set of options” because the race against the virus looks so different. He and Bubar showed that similar shifts in strategy could rely on other factors, such as different reproduction numbers and overall transmission rates.
Now, though, the debate over vaccination concerns not just which people to vaccinate but also how and when to do it. The two approved vaccines in the U.S. each require two doses to deliver their full 95% efficacy. But given how slowly those vaccines are being distributed, researchers have begun modeling other scenarios, including giving as many people the first dose of the vaccine as possible, rather than setting aside half of the available doses to absolutely ensure that people who receive their first dose will receive their second on schedule.
That strategy has the advantage of ramping up baseline protection in a population:The first dose confers only about 52% protection, which isn’t satisfactory by the usual vaccine standards but could slow the spread of infections enough to prevent more cases and deaths in the long run. But it’s also gambling on the likelihood that enough second doses will be available when they are needed. People who receive their second dose later than intended may never get the full measure of vaccine immunity, and some researchers worry that widespread delays in producing full immunity could give the virus more opportunities to mutate and “escape” a vaccine’s control.
SEIR models have helped to quantify that gamble. Assuming certain dose efficacies, waning efficacy between doses over time, and other factors, the models have shown that trying to deploy as many first doses as possible can avert around 25% more COVID-19 cases (and sometimes more) over eight weeks than setting aside half of available doses can. In fact, the researchers found that only in the worst-case scenario — if the first dose of the vaccine had a very low efficacy and if the vaccine supply chain collapsed — would setting aside half the doses for the future be the better alternative.
Ferrari points out that this trade-off is nothing new:He’s seen it in his own work on measles and meningitis outbreaks, and in colleagues’ work on cholera, yellow fever, polio and other diseases. The mathematical models are straightforward, he says, and they show that in the midst of an outbreak, the emphasis should always be on quickly vaccinating as many people as possible, even if it means sacrificing some of the efficacy of the vaccination campaign.
Such models have been instrumental in leading the U.K. — and now the U.S. — to adopt that plan. Perhaps they wouldn’t have if the virus hadn’t “just put on rocket boots,” as Larremore put it, or if the vaccine rollouts had happened more efficiently in the first place. But that’s why models have to take into account so many possibilities and uncertainties. (There are other open questions, too, like how long immunity will last, and whether COVID-19 will be a one-time crisis or a seasonal ailment like the flu, which will affect future decisions about how many vaccines to continue buying and how to prioritize them.)
“The math is simple,” Ferrari said. “Where the math meets the real world is where the complications come in.”
Going forward, there are still questions about human behavior to reckon with. Because of disabilities, poverty or other obstacles, some people may not be able to get to a vaccine distribution center, or they may be hesitant about getting a vaccine at all. As vaccines protect those most susceptible to COVID-19, “we’ll see mortality drop — along with the coming of spring and the opening of more of the outdoors to people,” Larremore said. “I think we’re going to see a lot of pressure put on officials to really reopen things.” People will start to act differently regardless. Will they get less careful or take more risks after getting a first dose or a second, or after seeing more and more people receiving vaccines? How will that affect transmission and subsequent vaccination and intervention strategies?
As with so many decisions that have characterized the COVID-19 pandemic, it’s still true that, while one strategy might have a bigger impact for all of society, it might not be beneficial to certain individuals — say, those who could get a significantly delayed second dose. It places at odds “the perspectives of the medical doctor who sees the patient in front of her,” Larremore said, “and the public health modeler who sees how things are shifting at a very broad scale.”
That’s something that all modelers have encountered. “I can type in these numbers, or see the results saying this number of people might die if [these are] the groups that we choose to vaccinate,” Bubar said. “Just looking at it as a coding simulation, it feels very impersonal. But then we turn on the news and we see the number of actual people that have died every day, and it’s obviously very personal and very shocking.”
That’s why she and Larremore have also tried to incorporate questions about fairness and ethics into their vaccine prioritization models, including a strategy of combining vaccine rollout measures with antibody test results, particularly in areas hit hardest by the virus.
Meanwhile, the pandemic has stolen attention from other health issues, for instance through disruptions of major health care services — including, in many countries, vaccination programs for other diseases. Ferrari has been analyzing how these disruptions will affect measles outbreaks around the world over the next couple of years. Some of his work has already helped to prompt Ethiopia to move ahead with planned measles vaccination programs and other health care services; he’s currently doing more modeling work to determine when and how other regions should resume those practices.
Looking Forward, Looking Back
Researchers expect to be dissecting what happened during the COVID-19 pandemic for years to come. They will comb through the massive numbers of models that were generated and try to account for what worked, what didn’t and why. But there are key lessons they are already taking away from the experience in preparation for the inevitable next pandemic.
One is that they should take advantage of new, potentially rich streams of data from cell phones and other sources, which can provide detailed information about people’s real behaviors. Another is that certain kinds of problems are most easily conquered by dividing them up among teams, sometimes spanning several disciplines.
To make that possible, Manrubia and other researchers have called for national and worldwide programs dedicated to epidemiological forecasting and pandemic science. “We need to undertake a global program, similar to those meteorological institutes that you have in all countries,” Manrubia said. “This is something that doesn’t exist at the epidemiological level. And we need that, at the world scale.”
Such a program might guide the development of extensive systems for data collection and sharing, as well as infrastructure for rapid testing, contact tracing and vaccine production. It could frame more coherent strategies for when and how to use certain types of models for optimal results. It could also establish networks for helping experts in diverse fields to connect, and it could offer protocols for integrating their areas of expertise into informed decision-making processes. The COVID-19 pandemic has broken ground for building those capabilities.
But the other crucial lesson of COVID-19 has been that epidemiologists need to communicate the proper uses and limitations of their models more effectively to decision-makers and the public — along with an appreciation of what the uncertainties in those models mean. The frustrating challenge is that researchers are often already offering these explanations, but the public and its representatives tend to want more certainty than science can provide. And when governments decide to disregard researchers’ best counsel and clutch instead at specious but popular policies, it isn’t clear what scientists can do about it.
Drake says he had hoped that U.S. policy leaders understood how measures like lockdowns could create time to formulate a national response to the pandemic. “I thought we did the right thing by locking down. And then we squandered [it]. We bought ourselves a month,” he said. “But we didn’t do anything with that time.”
Jewell is also outraged at what he called the “shambolic” U.S. response. “There really should be a national strategy:If you’re in an area with this level of community transmission, this is what your schools could do,” he said. Instead, “there is no plan. There is no strategy. Everyone — every campus and every school system — is on their own.”
He points accusingly at “the shocking performance of the CDC.” He doesn’t blame individual researchers there, but “somehow, politically, the CDC has been completely compromised,” he said. “We always used to turn to the CDC:‘Give us advice! What do we do?’ They were getting the best quality data.” But during this pandemic, that hasn’t happened. “They’ve given terrible advice.”
Drake recognizes that “it’s a policy decision, not a scientific one, as to what tradeoff you’re willing to accept in terms of the cost to the country and the number of lives lost — how many deaths could have been averted, what are we willing to pay for those.”
“But from my vantage point,” he continued, “much of the death and illness that we’ve seen in fact could have been prevented.” The models can warn us about fatalities to come, but we have to be willing to learn how to listen to them.